There has been an ongoing debate on the desirability and feasability of using human rights databases, more concretely the Cingranelli-Richards (CIRI) human rights dataset, to analyze trends in human rights. Neve Gordon and Nitza Berkovitch’s broadside attack on the alleged bias inherent in cross-national data being used in human rights research was quickly followed by Todd Landman’s critique of this allegation. Chad Clay, one of CIRI’s dataset principal investigators, then joined in to acknowledge some inherent weaknesses in using cross-national datasets but offered a qualified defense for using them.
My contribution to this debate focuses squarely on the inherent methodological weaknesses of the CIRI dataset from the perspective of a quantitative political scientist—weaknesses that are strong enough to merit a complete rethink in the way data are coded.
At first glance, the CIRI dataset offers an impressive array of operational variables, fourteen in total. They include variables on direct human rights violations (e.g., disappearances, torture), limitations on freedoms (of the press, assembly, etc.), and the protection of rights (such as worker’s rights). The coding manual (latest version 5.20.14) provides comprehensive explanations on how data were collected and coded.
What the CIRI dataset represents is a variation of the old Sovietologist’s content analysis of Soviet periodicals.
As a quantitative political scientist, however, the most noticeable features (or red flags, in my case) are the source and the coding of the data. Most of the data comes from a single source, the US State Department’s Human Rights reports. As a political economist I often use data from a single source (e.g., the IMF), however these data are actually cross-national because they are compiled from each IMF member country’s statistical agencies. In this sense, the CIRI dataset is not really a cross-national dataset; it is a single country’s cross-national evaluation, and the inherent bias in a single-country’s evaluation is worrisome. What the CIRI dataset represents is a variation of the old Sovietologist’s content analysis of Soviet periodicals, looking for clues in the Kremlin’s political decision-making. It may tell you something about the information being analyzed, but it also tells you a lot about who is doing the analysis.
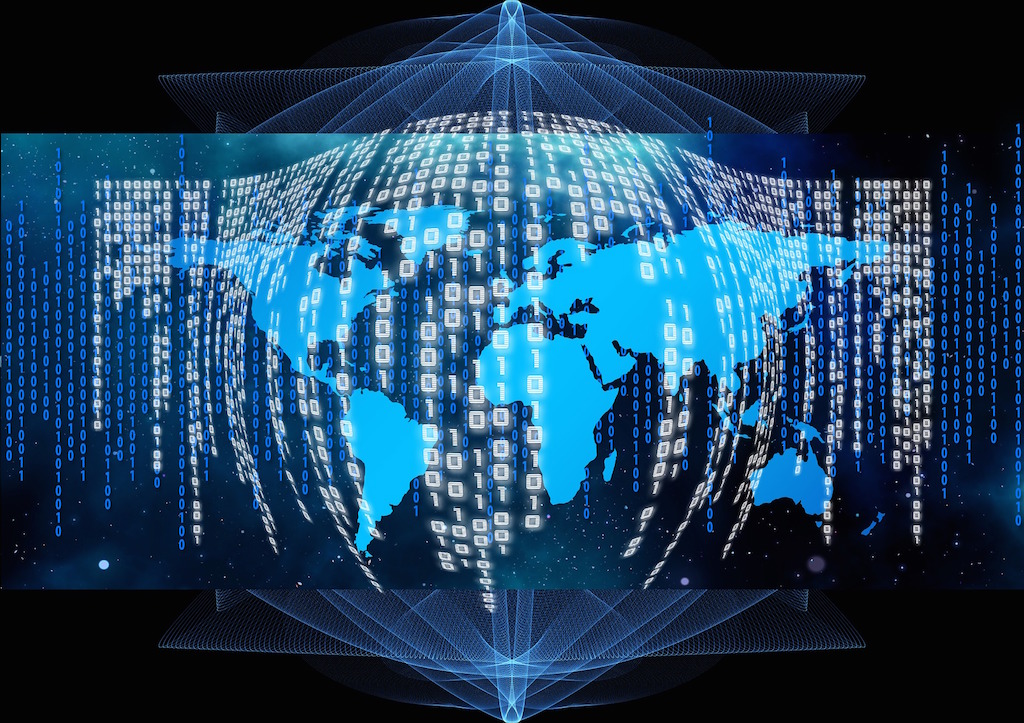
Flickr/Pixabay (Some rights reserved)
The CIRI dataset may tell you something about the information being analyzed, but it also tells you a lot about who is doing the analysis.
There is an inherent bias here that is difficult to overlook. The US State Department does not operate in a vacuum, it reflects US government policy which is not likely to be empirically neutral. To be fair, the CIRI dataset codebook makes mention that the data they collect from US State Department is cross-checked with other sources, but it is unclear where there may be a discrepancy between the State Department’s evaluation and alternative sources of information.
The other serious issue with the CIRI dataset is the type of coding. The coding scores for almost all variables range from 0 to 2. In not coding variables from a binary system of 0 to 1, it avoids having the pitfalls of building a dataset around dummy variables. However, having all variables range from 0 to 2—in effect making it an ordinal or spatial scale variable—is still way too narrow a range to be able to make meaningful conclusions. It is, in methodological terms, neither fish nor fowl. Ideally, an ordinal variable should range from 0-5, 0-10, or 0-100. CIRI’s coding scale of 0-2 means that 0 represents the complete absence or presence of an event (say electoral fraud), whereas the differentiator between 1 and 2 may mean a little or a lot. The absence of nuanced scale yields conceptually vague outcomes, both qualitatively and quantitatively.
There are numerous methodological problems that emerge from CIRI’s narrow coding scale. One of the fundamental expectations from working with parametric data is that it must have equal covariance within variables, namely that the individual values of variables change over time. CIRI’s dataset fails to meet these standards and its narrow coding scale presents additional problems. The first one is that there is little or no variation within each individual country scores. Moreover, there is little or no covariance within each variable. Countries that consistently violate human rights are likely to have the same score longitudinally and cross-sectionally; for many countries there is no longitudinal and cross-sectional variation in the data. From a quantitative perspective, the expectation is that there would be very serious econometric problems (e.g., multicollinearity, autocorrelation, and heteroskedasticity) that could provide highly distorted and biased results—perennial concerns to the quantitative political scientist. However, given how the variables overlap, full usage of the CIRI dataset is inherently structured to generate such statistically skewed results.
The second problem that emerges is from having such a narrow scale. If there is some quibbling with the presence of an undesirable event, such as the voter restrictions against African-Americans in the US (as raised by Gordon and Berkovitch), a country’s score could be devalued from having a perfect score (say a 0), to having a score of 1. If we take another example (e.g., complete freedom of the press), a country that may have a temporary gag on freedom of the press (say, for instance, to prevent the release of information during an ongoing response to a terrorist attack), would score a 1, rather than a 0. This expectation of complete perfection makes the CIRI dataset very vulnerable to challenges from qualitative researchers who may point out relatively minor problems as being determinative that a country does not have a completely clean human rights record. CIRI could transform some of their variables from a 0-2 scale to a more disaggregated scale. This can be easily accomplished by re-scaling the coding for some variables. The other, more attractive, option would be to convert the existing scale into fuzzy sets—subdividing a binary scale into several subcategories—thus enabling greater flexibility in the way that individual countries are coded. For instance, a fuzzy set for freedom of the press could be coded from 0 to 1, but within this scale there would be incrementally measured and qualitatively defined subunits. So, complete freedom of the press could be coded as a 1, whereas complete freedom of the press with some restrictions would be coded as .83, complete freedom of the press with substantial restrictions would be coded as .66 and so on. This recoding could provide more internal nuance to each of the variables.
In general, I am very supportive of the effort to analyze changes in human rights protections from a cross-national perspective. I believe that dataset initiatives, like CIRI, can help us develop a more nuanced understanding about such trends. However, at present, the CIRI dataset suffers from significant methodological problems that may make it useless for any meaningful statistical analysis.
*** This text was updated on April 5, 2017 to correct for some of the factual errors noted in comments below.